A CONVERSATION WITH
Charles Dunnett, SSC 1986 Gold Medalist
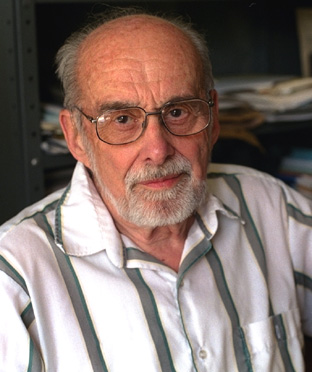
Charlie Dunnett, McMaster, May 2002
photo by Peter Macdonald
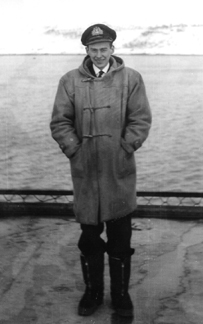
Murmansk, April 1944
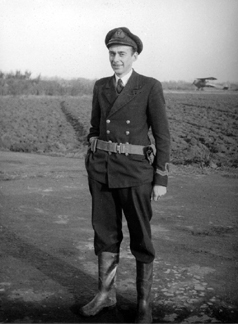
Belgium, October 1944
Charles W. Dunnett was born in 1921 in Windsor, Ontario. He graduated in 1942 with a BA in Mathematics and Physics from McMaster University. He served in the Royal Navy during World War II, and was awarded an MBE for work on radar. Returning to Canada, he obtained an MA in Mathematics at the University of Toronto in 1946. Following two years at Columbia University and a year spent teaching at the New York State Maritime College, in 1949 he joined the Food and Drug Laboratories of the Department of National Health and Welfare in Ottawa as a biometrician. He spent 1952-53 on leave at Cornell University. In 1953 he accepted a position as statistician at Lederle Laboratories, a division of American Cyanamid Company, and remained with them until 1974, when he was appointed Professor of Clinical Epidemiology and Biostatistics in the Health Sciences Faculty at McMaster University. He was chairman of the Applied Mathematics Department at McMaster from 1977 to 1979, and subsequently a member of the Department of Mathematical Sciences. He was awarded the title of Professor Emeritus in 1987 in the Departments of Clinical Epidemiology and Biostatistics and Mathematics and Statistics.
Professor Dunnett obtained his doctorate in 1960 working with Professor D.J. Finney at Aberdeen University. He is a Fellow of the American Statistical Association and a Member of the International Statistical Institute. He served as President of the Statistical Society of Canada in 1982. In 1986 the Society awarded him its Gold Medal for his contributions to Statistics.
The following excerpts from a conversation recorded July 5, 1988 at McMaster University originally appeared in Liaison Vol. 3, No. 1, November 1988.
L = Liaison, D = Dunnett.
L. Would you start by telling us about your radar work, for which you were awarded the MBE?
D. When I graduated from here [McMaster University] with a Bachelor’s degree I went right into the service, and used the electronics background from my physics training to enlist as a radar officer. I was on loan to the Royal Navy. I went to England and took some training courses, and then went to a research establishment where they were developing radar equipment for aircraft in the R.A.F. and the Fleet Air Arm of the Navy. I spent about six months working with the research people on a new type of radar for detecting submarines and other vessels on the surface of the sea, and then went out into the field, so to speak, with the radar equipment. First to a training squadron, where they trained the air crew to operate the radar. Then I spent a year and a half with the first operational Fleet Air Arm squadron to use the radar. We were on board a carrier for some months, patrolling in the Atlantic and on the Murmansk convoy run, and then before D-Day we were disembarked from the carrier and stationed on an RAF airfield in the south of England, where the squadron supported the D-Day landings by searching for and attacking enemy shipping in the English Channel at night. We moved over to Belgium when Northern Europe was liberated and operated from airfields there until the end of the war in Europe. So that was my naval career. I was in charge of the maintenance of the radar, and there were lots of new problems with getting the radar in service. We were the first ones to use it, so the first ones to discover the problems. My job was to find solutions to the technical problems if I could, and consult with the research people in England when their help was needed.
L. Did you go back to university right after the war?
D. Yes. I was scheduled to transfer to the Pacific area, but when the war suddenly ended I decided to do a Master’s degree in mathematics at the University of Toronto. One of the courses I took happened to be a statistics course, which fascinated me, and I decided that statistics was what I was interested in doing. There was another student at Toronto that year, Colin Blyth, who was also a major influence on my going into statistics, besides the course I was taking. His plan after finishing his master’s degree at Toronto was to go to Iowa State University, which had of course Snedecor, Cochran and Gertrude Cox at that time. He had applied to go to Iowa State so I did also. We were both accepted, planning to go there, when we learned that Cochran and Gertrude Cox were leaving to go to North Carolina. For all we knew there was no one left at Iowa State! Actually that was not the case, but Colin transferred to the University of North Carolina and I applied to go to Columbia University where Wald and Wolfowitz were.
I stayed at Columbia in the Department of Mathematical Statistics for two years. I was planning to do my doctorate there, but didn’t do the thesis work. Took all the course work. Passed the qualifying examination. Then decided I had studied enough theory and wanted some practical experience. After one year teaching mathematics and physics at the New York State Maritime College in the Bronx, I went to Ottawa and joined the Food and Drug Laboratories as a biometrician.
That was an interesting period for me because I became exposed to lots of applied problems in statistics. We had problems in sampling, for instance. I had a couple of trips to Halifax where they took samples of food products coming off the ships. I worked with Dr J.W. Hopkins who was a biometrician at the National Research Council. He had developed an interesting theory for sampling these types of products. The physical characteristics of the problem required a two-stage sampling design, because the food products — primarily nuts, dates or figs — were packed in containers such as boxes or bags. The primary sampling unit had to be the container and a sample of containers was selected, and then within each container a secondary sample of items was selected for examination. Each individual item was defective if it was contaminated, for example with insect infestation or mould, and the problem was to determine the proportion defective in the shipment. The theory was based on assuming that within a container the defectives were randomly distributed, in an infinite population, so that if you had a sample of a certain size from only one container the binomial distribution was applicable. But then the binomial parameter could vary from container to container in the shipment, and they had data to show that this was the case. In Dr Hopkins’ model the binomial parameter varied in a beta distribution and that produced what he called the negative hypergeometric distribution but has since become known as beta-binomial.
L. Did the use of this distribution involve much computation?
D. Yes, there were heavy computations involved in estimating the parameters of this negative hypergeometric distribution. We used moment estimates of the parameters and then developed an acceptance sampling procedure for the shipments (1).
Another major problem was biological assay. They did a lot of experiments to determine potencies of drugs relative to a standard drug. Probit analysis was used, and again the computations were pretty heavy. The standard procedure was that the laboratory person would spend one day in the laboratory doing the experiment, taking the observations on the animals. The next day he’d spend at his desk calculator, doing the calculations needed to determine the potency estimates.
L. When did you first become involved in academic research?
D. I left the Food and Drug Laboratories on a one-year leave of absence to go to Cornell University, where I was invited to work with Bob Bechhofer and Milton Sobel, who had been graduate students at Columbia at the same time I was there. Bob Bechhofer had a research grant to work on selection procedures. I had a very productive year there. We were officially in the Mathematics Department, but actually our offices were in another building, in Warren Hall, which was in the agricultural area. Walt Federer and Doug Robson had their offices there and we really saw as much of them as we did of Jack Kiefer and others in the mathematics faculty.
L. What were the selection procedures you were working on?
D. The main application was selecting the best treatment: for example, selecting the population with the largest population mean. The idea was that if in an experiment several treatments are being compared, you could do an analysis of variance and test the hypothesis that all the means are equal with the F test and so on, but in many applications the null hypothesis of no treatment differences doesn’t answer the real problem. The real problem is to determine which is the best of the treatments. We wanted a procedure that would guarantee a specified probability of correctly selecting the best population mean. Of course this probability depends upon the configuration of the population means, which is unknown. If the true population means are very close to each other, you can’t guarantee a very high probability of correctly selecting the highest one. But if the true population means are very close together, it’s not so important that the best one be correctly selected. It’s only important if the best one is more than a specified amount better. You specify the difference that’s important to be able to detect. Then you can determine what size of experiment is required. Bob Bechhofer had formulated the selection problem in this way, which has become known as the “indifference zone” approach, and developed the necessary theory for the case of normal populations with a common, known variance. This was described in his 1954 Annals paper (2).
In extending his methods to the unknown variance case we encountered or formulated the multivariate Student’s t distribution. I suppose that was the main theoretical contribution made during the year I spent at Cornell. There were two papers published in Biometrika (3), (4). The multivariate distribution was defined there, though mostly we dealt with the two-dimensional case because again, all we had were desk calculators to work with. Also, we considered that two dimensions were a major step forward from one!
L. The Cornell experience seems to have led to a decision to leave the Food and Drug Laboratories permanently.
D. I decided that I wanted to move to the United States, only because there were more statisticians around in the U.S. I had an offer of a position at Lederle Laboratories, a pharmaceutical company in Pearl River, New York. There was a small statistical group there already. Dr Frank Wilcoxon was head of the group. The interesting thing is that immediately on joining Lederle I found another application for the multivariate Student’s t distribution that I think may have turned out to be even more important than the selection applications, namely comparing treatments with a standard treatment. In fact, multiple comparison methods had just come into use at that time. Scheffé’s paper (5), which would be published in Biometrika, was known to quite a few people. Tukey’s work on multiple comparisons was known, and Duncan’s work was known. The day I started work at Lederle, Dr Wilcoxon went through with me some problems they were working on, and he mentioned this problem of multiple comparisons between treatments and a control treatment as one which Tukey and Scheffé had not dealt with. He showed me a paper by Roessler in the Journal of Horticultural Science, predating Tukey and Scheffé, on this very problem. The method Roessler had developed was not rigorous, in that it ignored the correlations between the comparisons of treatment vs. control that are present if the same control is used in all the comparisons. I took Roessler’s method and saw that if the correlations were taken into account you were led to exactly the same multivariate Student’s t distribution that we had developed the year before at Cornell University. If all the sample sizes are equal for the treatments and the control, and if the variances are homogeneous, then the correlations are all 0.5, because of the presence of the same control mean in all of the comparisons. In the selection problem the same correlation 0.5 comes in because all the comparisons are with the best treatment.
L. The 1955 JASA paper (6) which gave critical values for the most extreme difference between treatment and control means, and the 1964 Biometrics paper (7) which refined and extended the method, are still very widely cited in the scientific literature.
D. Yes, they’ve been cited a lot.
The second major problem that I became interested in at Lederle Laboratories was drug screening, the problem of testing chemicals for biological activity. A drug company will have a large file of chemical compounds that they or others have developed, and the question is, do any of these compounds have any biological activity that might eventually make them useful as drugs for treating disease? The first step is to do a quick test in animals of a large number (20 or 30) of the compounds, then select a subset for further testing, accepting or rejecting the others. I had taken a course from Abraham Wald at Columbia University on sequential analysis, and was interested in finding applications for sequential methods. In drug screening, for a particular drug, the typical experiment would be completed in a time frame of a week or two, where the animal is treated with the drug, the results are observed, and then the experimenter can decide whether to repeat the test. It is actually feasible to repeat the test several times, in successive experiments, before coming to a decision on activity or non-activity. There were a number of applications that I worked on at Lederle, setting up sequential procedures for deciding: accept the drug, reject the drug, or test it again.
In 1957 I learned that Dr O.L. Davies of I.C.I. Pharmaceuticals was scheduled to present an invited paper (8) on drug screening at the ISI meetings in Stockholm. Lederle’s management considered this to be an important enough reason for me to travel to Sweden to attend the conference. For me, it was doubly fortunate that Professor D.J. Finney also attended and presented a paper (9) on similar problems in the agricultural area: selection of new plant varieties. This led to an exchange of correspondence between us, resulting in my going to Aberdeen the following year with my family on a two-year educational leave from Lederle to work with Professor Finney on a D.Sc. thesis.
L. What was the department like at Aberdeen?
D. Professor Finney was head of the Department of Statistics. He was also head of the Agricultural Research Council’s Unit of Statistics, which was based at Aberdeen. He had the two groups, but actually they were pretty well merged: faculty members worked on A.R.C. problems, and A.R.C. members taught courses in the Department of Statistics. Michael Sampford was there, as well as Bill Brass, and Peter Fisk. Richard Cormack was working on his doctorate at that time. So was Robert Curnow, and he and I worked very closely together because he was working on plant selection problems which involved a similar theory to those I was working on.
L. Was the paper (10) which was read before the Royal Statistical Society based on your thesis?
D. Well, that was part of it. I went there to work on a thesis on drug screening, and at the same time I continued some work on selection. That R.S.S. paper was the selection part of it. I worked on a different model from what we had worked on at Cornell. It was a sort of Bayesian model where prior information was assumed about the population means. So it turned out that the thesis was divided between the two problems of drug screening and selection. The screening part (11) was published in the proceedings of a conference on pharmacological statistics held at the University of Leiden in the Netherlands.
L. Again there was a good deal of computation involved. I have the impression you must enjoy working out numerical methods.
D. Yes, I enjoy computing. We got our first computer at Lederle in 1957. It was a Royal McBee LGP-30, and had a magnetic drum memory. We eventually upgraded it to 8000 words, but I think it was about half that to start with. All computations had to be programmed in machine language. It was actually fairly easy to program. There were sixteen different operations, like add, subtract, store… each operation followed by a four-digit address, to indicate where the number was stored. Programs and data had to be punched on a long roll of paper tape. That was fun. You had to make sure the paper tape didn’t get tangled up. Our offices were on the sixth floor, in the tallest building at Lederle, so to unravel the tapes we just tossed them out the window. We had six floors to let them unroll down.
L. Things have come a long way since then.
D. I’m afraid so.
L. Recently using more modern devices you published a couple of papers in JASA (12, 13) on pairwise multiple comparisons which were very interesting.
D. I guess the main point of those papers was that they showed by computer simulation that the studentized range method developed by Tukey in the early 1950’s for doing all pairwise treatment comparisons could be extended to cases where the treatment groups had unequal sample sizes. This had been proposed years earlier, proposed by Tukey himself, but there was no rigorous justification for it. In Tukey’s method with equal sample sizes you’d use the studentized range point times s/sqrt(n) as the allowance or the critical value for comparing any one treatment with another treatment in the group. If the treatments have unequal sample sizes, one method which was proposed was to replace s/sqrt(n) with s*sqrt(1/n1 + 1/n2)/sqrt(2), the standard error of the difference between two means, divided by sqrt(2) to make it comparable. This was an intuitive method only: it wasn’t known whether the joint confidence coefficient was 1-a.
There were a number of other methods proposed that did have the mathematical justification that it could be shown their joint confidence coefficient became greater than the nominal value as the sample sizes became unequal. But my computer simulation experiments showed that the confidence intervals based on the natural extension of the studentized range, which of course were narrower, had this property also. And that was since mathematically proved for the equal variances case in a paper by Hayter in the Annals of Statistics (14). In the unequal variance case I think computer simulation is the only possible way of providing justification for methods analogous to the studentized range. Another paper (15) dealt with robust methods for multiple comparisons.
L. Was it the medical program which made you decide to come back to McMaster?
D. Yes, primarily. From my pharmaceutical work I was interested in medical applications.
L. Your 1977 Biometrics paper with Gent (16) on equivalence testing was motivated by medical applications.
D. Yes, some medical studies are carried out with the aim of showing that the usual null hypothesis of equal treatment effects is true rather than false. It was Mike Gent’s idea that this required a change in the usual philosophy of hypothesis testing. There have been other papers published on a similar theme, but ours was one of the first, I believe.
L. What are you working on these days?
D. I’m just getting back to drug screening now. I have some ideas for further work in that area I hope to work on next year. Still working on multiple comparisons, and have a paper with Bob Bechhofer at Cornell and Ajit Tamhane at Northwestern University that I’ll be giving at the Biometric Conference in Belgium later this month. This is on a method for doing multiple comparisons between treatments and a control, and we’re interested in joint confidence interval estimation for each treatment vs. the control treatment. But the aspect that’s different is that not all of the treatments have a sufficiently high mean to be of interest, so a preliminary experiment is done to try to weed out the inferior treatments, and then the main experiment is done on those that survive the first phase. We examine under which situations it’s more efficient to use both phases to determine joint confidence intervals for the comparisons of interest, rather than using the first phase merely to eliminate inferior treatments and the second phase to determine the confidence intervals.
L. You also have some connection with the University of Swansea in Wales, haven’t you?
D. No official connection now, but I spent two research leaves there from McMaster and had honorary appointments as a visiting professor. My wife and I frequently visit the Swansea area, and the Department of Management Science and Statistics at the university and has been very generous in providing me with facilities such as computer time and work space when I am there.
L. Thank you, Professor Dunnett.
References
- Dunnett, C.W. and Hopkins, J.W. (1951). Symposium on Bulk Sampling, Spec. Tech. Pub. No. 114, Amer. Soc. for Testing Materials, 13-18.
- Bechhofer, R.E. (1954). Ann. Math. Statist., 25, 16-39.
- Bechhofer, R.E., Dunnett, C.W. and Sobel, M. (1954). Biometrika, 41, 170-176.
- Dunnett, C.W. and Sobel, M. (1954). Biometrika, 41, 153-159.
- Scheffé, H. (1953). Biometrika, 40, 87-104.
- Dunnett, C.W. (1955). J. Amer. Statist. Assoc., 50, 1096-1121.
- Dunnett, C.W. (1964). Biometrics, 20, 482-491.
- Davies, O.L.(1958). Bull. I.S.I., 36, 226-241.
- Finney, D.J. (1958). Bull. I.S.I., 36, 242-268.
- Dunnett, C.W. (1960). J. Roy. Statist. Soc. B , 22, 1-40.
- Dunnett, C.W. (1961). Quantitative Methods in Pharmacology (H. de Jonge, ed.), North–Holland, 212-231.
- Dunnett, C.W. (1980). J. Amer. Statist. Assoc., 75, 789-795.
- Dunnett, C.W. (1980). J. Amer. Statist. Assoc., 75, 796-800.
- Hayter, A.J. (1984). Ann. Statist., 12, 61-75.
- Dunnett, C.W. (1982). Comm. Statist. A, 11, 2611-2629.
- Dunnett, C.W. and Gent, M. (1977). Biometrics, 33, 593-602.